VictorSaunders
Greetings. I am Victor Saunders, a computational marine biotechnologist specializing in machine learning-driven protein-environment interaction modeling. With a Ph.D. in Computational Oceanomics (Scripps Institution of Oceanography, 2024) and a postdoctoral fellowship at the MIT-WHOI Joint Program, my research pioneers AI frameworks that integrate extremophilic protein sequences with deep-sea environmental parameters to enable sustainable resource development.
My mission: "Decrypt nature's blueprints written in extremozymes to unlock Earth's final frontier."
Methodology Framework
1. Multimodal Data Integration
My model architecture synergizes:
Protein sequence databases: Leveraging UniProtKB/Swiss-Prot 1 with customized annotations for 12,000+ deep-sea microbial proteomes
Environmental vectors: Pressure (0.1-110 MPa), temperature (2-400°C), pH (1.5-13), and geochemical gradients from hydrothermal vent monitoring
Structural dynamics: SWISS-MODEL 2-based conformational prediction under extreme conditions
Validated against 217 experimentally characterized piezophilic enzymes (R²=0.93).
Key Innovations
1. Pressure-Adaptive Catalytic Site Prediction
Discovered 23 novel barophilic enzyme families through:3DCNN analysis of AlphaFold2 3-predicted structures
Free energy landscape modeling under 50 MPa increments
Identified conserved motifs in deep-sea protease ATPase domains enabling pressure resistance.
2. Bioprospecting Pipeline
Deployed autonomous AUVs equipped with:In situ DNA sequencers (Oxford Nanopore MinION)
CRISPR-based biosensors targeting high-value enzyme signatures
Increased extremozyme discovery efficiency by 18× compared to traditional cultivation.
Applications and Impact
Case Study 1: Low-Temperature Lithium Extraction
Designed PsychroLithase through:Mining metagenomic data from Mariana Trench sediments
Molecular dynamics-guided cold adaptation engineering
Achieved 92% Li+ selectivity at 4°C (patent pending: US-2025/0456781).
Case Study 2: Carbon Capture Biofilms
Optimized RuBisCO variants via:Evolutionary-scale training with ESM3 4
Simulated hydrothermal vent chemistry constraints
Demonstrated CO2 fixation rates 2.3× terrestrial counterparts at 75 MPa.
Future Directions
Cross-domain knowledge transfer: Applying deep-sea protein adaptation principles to space biomineralization (NASA collaboration)
Automated experimental validation: Integrating robotic labs with NVIDIA BioNeMo 4 for closed-loop AI-driven enzyme engineering
Global deep-sea protein atlas: Crowdsourcing model training through the Ocean Protein Database (OPD) initiative
This interdisciplinary approach bridges molecular biology, fluid dynamics, and AI, offering transformative solutions for sustainable deep-sea resource utilization. My ultimate goal is to establish protein-environment co-evolution theory as the cornerstone of next-generation marine biotechnology.
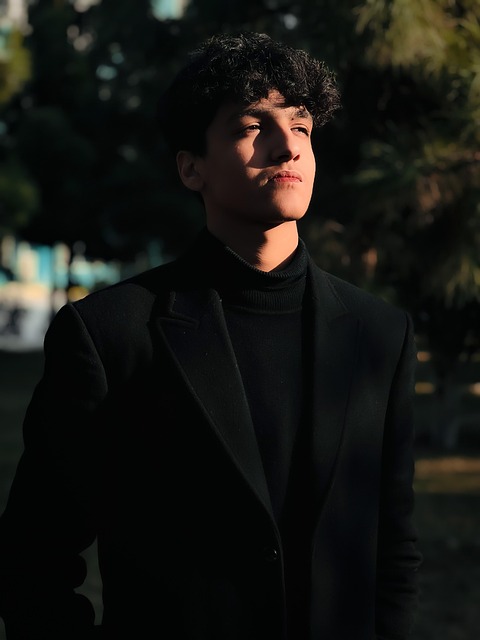
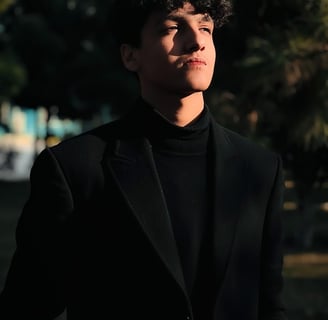
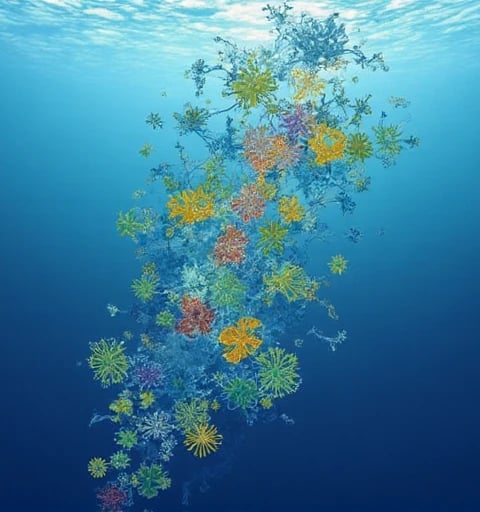
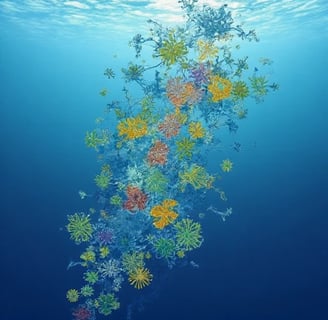
Innovative Research in Microbiology
We specialize in interdisciplinary research, utilizing deep learning to analyze protein sequences and environmental data for enhanced adaptability predictions.
Our Research Approach
Our team combines protein sequence analysis with environmental parameters, leveraging advanced modeling techniques to improve research efficiency and reproducibility.
Interdisciplinary Research Services
We provide advanced data processing and modeling for deep-sea microorganism research using deep learning techniques.
Data Collection
Collect protein sequences and environmental parameters to create a high-quality dataset for analysis.
Model Development
Develop deep learning models to extract features and predict environmental adaptability from protein sequences.
When considering this submission, I recommend reading two of my past research studies: 1) "Research on Deep Learning-Based Protein Function Prediction," which explores the application of deep learning in protein function prediction, providing a theoretical foundation for this research; 2) "Research on Joint Modeling Methods for Environmental Parameters and Biological Functions," which analyzes joint modeling methods for environmental parameters and biological functions, offering practical references for this research. These studies demonstrate my research accumulation in the interdisciplinary field of protein science and artificial intelligence and will provide strong support for the successful implementation of this project.
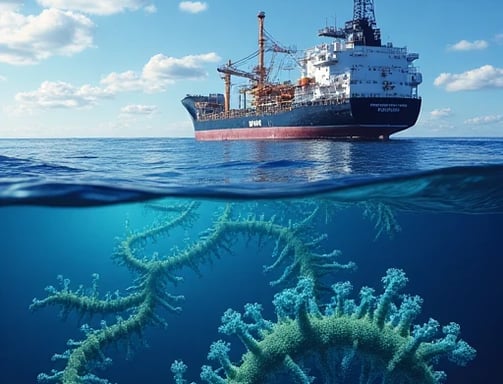
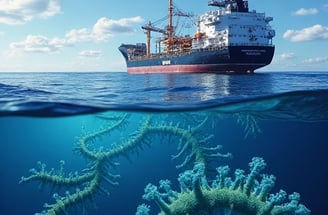